Breaking Down ColabFold and AlphaFold 2: Which is Right for You?
The differences between ColabFold and AlphaFold 2, two groundbreaking tools in protein structure prediction. Learn about their features, applications, strengths, and how they cater to different needs in the scientific community.
AI/FUTUREHEALTH/DISEASEEDUCATION/KNOWLEDGEMODERN DISEASES
Sachin K Chaurasiya
2/4/20255 min read
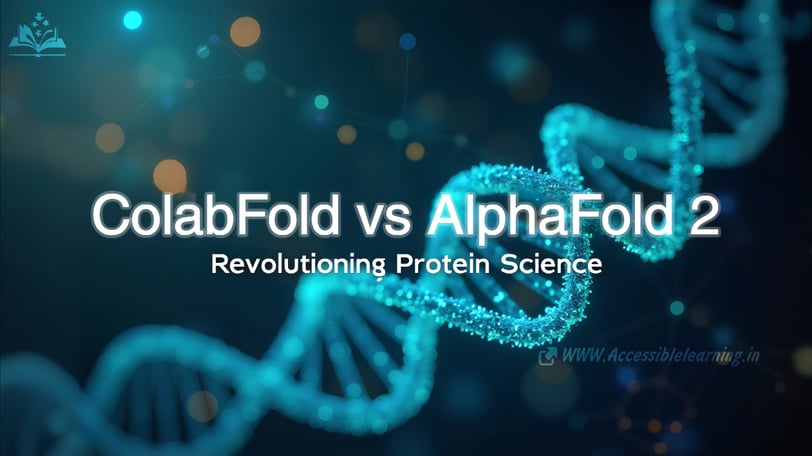
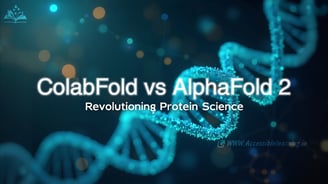
In recent years, advances in protein structure prediction have revolutionized the fields of biology and biotechnology. Among the most notable tools are AlphaFold 2, developed by DeepMind, and ColabFold, an open-source project inspired by AlphaFold but tailored for accessibility and speed. While both tools aim to predict protein structures with exceptional accuracy, they differ in methodology, user accessibility, computational requirements, and target audiences. This article explores these differences and highlights the unique features of each platform.
AlphaFold 2
AlphaFold 2, a brainchild of DeepMind, debuted in 2020 as a game-changer in protein structure prediction. It achieved unprecedented accuracy in the CASP14 (Critical Assessment of Protein Structure Prediction) competition, outperforming all other methods by predicting near-experimental accuracy structures for a majority of proteins. AlphaFold 2 uses deep learning and attention mechanisms, focusing on both evolutionary information and spatial relationships to achieve its remarkable predictions.
AlphaFold 2's algorithm integrates multiple inputs, including sequence alignments, predicted inter-residue distances, and structural templates, to construct high-confidence protein models. This has not only accelerated fundamental biological research but also opened avenues in drug discovery and synthetic biology.
ColabFold
ColabFold is an open-source alternative based on AlphaFold 2 but optimized for broader accessibility. Developed by researchers and hosted on Google Colab, ColabFold integrates features from AlphaFold 2 and RoseTTAFold while emphasizing speed and ease of use. It allows researchers with limited computational resources to predict protein structures using cloud computing, democratizing access to high-quality predictions.
ColabFold leverages faster methods for generating multiple sequence alignments (MSAs) and uses tools like MMseqs2 to enhance efficiency. It also provides pre-trained models and pre-computed MSAs, reducing runtime significantly. This makes it a practical solution for users with limited expertise or computational power.
Key Differences
Accessibility
AlphaFold 2: Requires substantial computational resources to run locally. Users must install the AlphaFold software, set up dependencies, and ensure access to GPUs with high memory. This setup makes AlphaFold 2 challenging for researchers without access to advanced computational infrastructure.
ColabFold: Hosted on Google Colab, it eliminates the need for local installations. Users can leverage free or paid Colab accounts, making it an ideal choice for researchers and educators with limited resources. The user-friendly interface streamlines the prediction process.
Computational Efficiency
AlphaFold 2: While highly accurate, AlphaFold 2’s predictions are computationally intensive. A single protein prediction can take hours to complete, depending on the size of the protein and the computational power available.
ColabFold: Designed for speed, ColabFold introduces innovations like faster multiple sequence alignment (MSA) methods and optional use of precomputed MSAs. This optimization reduces runtime significantly, often completing predictions in minutes.
Accuracy
AlphaFold 2: Known for its unparalleled accuracy, AlphaFold 2 consistently delivers results comparable to experimental methods like X-ray crystallography and cryo-electron microscopy for many proteins. It’s widely regarded as the gold standard in structure prediction.
ColabFold: While slightly less accurate than AlphaFold 2 in some cases, ColabFold’s predictions remain highly reliable. Its speed and accessibility make it a valuable tool for preliminary predictions and large-scale projects.
Ease of Use
AlphaFold 2: Requires expertise in bioinformatics and software installation. Users must manage dependencies, databases, and compute configurations, which can be daunting for non-specialists.
ColabFold: Simplifies the process with a Google Colab notebook interface. Users only need a Google account and some basic knowledge of running Jupyter notebooks, making it significantly more approachable for non-experts.
Features and Customization
AlphaFold 2: Offers a rigid structure with minimal customization options. It’s optimized for high accuracy but doesn’t provide extensive flexibility for user-defined parameters.
ColabFold: Includes advanced features like customizable MSAs, batch processing, and compatibility with AlphaFold’s and RoseTTAFold’s models. This flexibility caters to users with diverse needs, from simple predictions to complex analyses.
Applications and Use Cases
AlphaFold 2
Drug Discovery: Supports pharmaceutical research by elucidating protein-ligand interactions.
Basic Research: Enables biologists to understand protein functions and interactions.
Structural Biology: Complements experimental techniques to resolve challenging structures.
Enzyme Design: Facilitates the engineering of enzymes for industrial and medical applications.
Understanding Disease Mechanisms: Offers insights into the structural basis of diseases caused by protein misfolding or mutations.
Strengths
Industry-leading accuracy.
Widely validated by the scientific community.
Comprehensive database of predicted protein structures (via AlphaFold Protein Structure Database).
Limitations
High computational requirements.
Limited accessibility for non-specialists.
ColabFold
Education: Facilitates teaching protein structure prediction in classrooms.
Small Labs: Empowers researchers in resource-constrained environments.
High-Throughput Studies: Ideal for large-scale predictions due to their speed and flexibility.
Collaborative Projects: Enables distributed teams to work together seamlessly without the need for high-performance local infrastructure.
Sequence Mutational Analysis: Quickly predicts the structural impact of mutations in protein sequences.
Strengths
Easy to use and accessible via the cloud.
Faster predictions with lower computational demands.
Flexibility in input and model options.
Supports community-driven improvements and customization.
Limitations
Slightly reduced accuracy compared to AlphaFold 2.
Dependence on Google Colab’s infrastructure, which may have runtime limits for free users.
Scalability
AlphaFold 2: Designed for intensive, high-stakes research, AlphaFold 2’s scalability is constrained by the availability of powerful computational resources. It is less suited for batch processing or large-scale studies without access to high-performance clusters.
ColabFold: Its cloud-based infrastructure supports scalability, allowing users to run multiple predictions simultaneously in batch mode, making it ideal for projects requiring rapid, large-scale analysis.
Community and Updates
AlphaFold 2: Regularly updated by DeepMind, the tool remains at the cutting edge of accuracy and methodology. However, its closed-source nature limits community-driven contributions.
ColabFold: Thrives on community input and improvements. Its open-source nature encourages collaboration, ensuring continuous innovation and customization.
Integration with Other Tools
AlphaFold 2: Primarily a standalone application, with limited built-in integration capabilities.
ColabFold: Compatible with other bioinformatics tools, enabling users to integrate predictions into workflows involving molecular dynamics, docking studies, or mutational analyses.
Which One Should You Choose?
Choose AlphaFold 2 if: You have access to high-performance computing resources and require the most accurate predictions for critical research projects.
Choose ColabFold if: You value ease of use, speed, and accessibility, especially if you lack the infrastructure to run AlphaFold 2 locally.
For collaborative or educational settings, ColabFold provides an unmatched advantage due to its simplicity and speed. Meanwhile, AlphaFold 2 remains the go-to solution for researchers requiring the highest level of accuracy.
Both AlphaFold 2 and ColabFold are remarkable tools that have reshaped protein structure prediction. AlphaFold 2 sets the benchmark for accuracy, while ColabFold democratizes access to these predictions, making them feasible for a wider audience. The choice between the two depends on your specific needs, resources, and expertise.
As protein structure prediction continues to evolve, tools like AlphaFold 2 and ColabFold will remain indispensable, driving breakthroughs in biology, medicine, and beyond. By leveraging these technologies effectively, researchers can unlock new insights into the molecular machinery of life and push the boundaries of scientific discovery.
Subscribe to our newsletter
All © Copyright reserved by Accessible-Learning
| Terms & Conditions
Knowledge is power. Learn with Us. 📚