Protein Science Redefined: Inside RoseTTAFold by Baker Lab
The revolutionary impact of RoseTTAFold, a groundbreaking AI-driven tool from the University of Washington's Baker Lab. Learn about its origins, technology, applications in drug discovery, structural biology, and more, along with its potential to transform protein science.
HEALTH/DISEASEAI/FUTUREEDUCATION/KNOWLEDGEEDITOR/TOOLS
Sachin K Chaurasiya
1/29/20255 min read
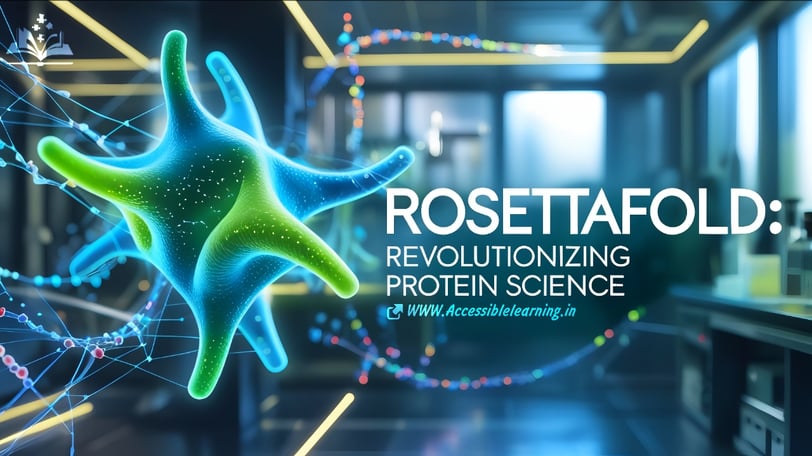
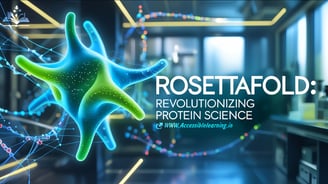
In the rapidly evolving landscape of structural biology, protein folding has remained one of the most significant scientific puzzles. The ability to predict protein structures with precision can unlock transformative breakthroughs in medicine, biotechnology, and bioinformatics. Among the remarkable contributions in this field, RoseTTAFold, developed by the University of Washington's Baker Lab, stands out as a groundbreaking innovation.
This article delves into the origins, technology, applications, and significance of RoseTTAFold in advancing our understanding of protein science.
The Origins of RoseTTAFold
David Baker’s lab at the University of Washington has been a pioneer in protein modeling and design. Their earlier creation, Rosetta, was a powerful tool that contributed significantly to computational protein design. However, challenges like the complexity of protein structures and the sheer scale of computational resources required for accurate predictions necessitated newer methods.
In 2021, Baker Lab introduced RoseTTAFold, an AI-driven deep-learning tool designed to tackle these challenges with greater efficiency. Released in the wake of the revolutionary AlphaFold from DeepMind, RoseTTAFold brought complementary capabilities, showcasing its unique strengths and collaborative potential in the protein-folding landscape.
The Technology Behind RoseTTAFold
At the core of RoseTTAFold lies a three-track neural network architecture, designed to process and integrate three types of input data simultaneously:
Protein Sequences: The amino acid sequence of a protein is the starting point for structure prediction.
Pairwise Interaction Information: RoseTTAFold identifies potential interactions between amino acids in the sequence.
3D Coordinates: It computes three-dimensional spatial relationships between amino acids.
This innovative approach enables the model to simultaneously refine sequence alignments, inter-residue relationships, and spatial arrangements in an iterative process. The result is a highly accurate 3D protein structure.
Unlike traditional computational methods that require extensive resources, RoseTTAFold’s design leverages AI to make structure prediction faster and more accessible. This democratization of protein structure prediction has profound implications for researchers globally.
Key Features and Strengths
High Accuracy: While not as precise as AlphaFold in certain benchmarks, RoseTTAFold excels in multi-domain protein predictions and protein-protein interaction modeling.
Open Accessibility: Baker Lab’s commitment to open science is evident in making RoseTTAFold freely available. This encourages collaboration and accelerates research worldwide.
Multi-Tasking Ability: The model’s ability to predict the structure of protein complexes, including interactions between different chains, makes it highly versatile.
Reduced Resource Dependency: RoseTTAFold is computationally efficient, requiring fewer resources compared to many traditional methods, which broadens its usability in labs with limited computational power.
Support for Complex Proteins: RoseTTAFold’s ability to handle multi-domain proteins and predict their folding pathways sets it apart as a robust tool for studying intricate biological mechanisms.
Collaborative Integration: RoseTTAFold is often used alongside experimental methods like cryo-electron microscopy (cryo-EM) to refine and validate predictions.
Applications of RoseTTAFold
Drug Discovery and Design
Accurate protein structure predictions are crucial for understanding how drugs bind to targets. RoseTTAFold facilitates the identification of novel therapeutic molecules by modeling protein-drug interactions.
Understanding Disease Mechanisms
Misfolded proteins are implicated in diseases like Alzheimer’s, Parkinson’s, and cystic fibrosis. RoseTTAFold aids in studying these misfolded proteins, potentially leading to new treatments.
Synthetic Biology
In synthetic biology, researchers design new proteins for specific purposes, such as enzymes for industrial applications. RoseTTAFold accelerates this process by providing accurate templates for protein design.
Antibody Design
With the rise of immunotherapy and antibody-based treatments, RoseTTAFold helps researchers predict the structure of antibodies and their interaction with antigens, optimizing their therapeutic efficacy.
Academic Research
The tool has empowered researchers in structural biology to tackle complex problems that were previously insurmountable due to computational or resource limitations.
Protein-Protein Interactions
Understanding how proteins interact with one another is critical in cellular biology and signal transduction studies. RoseTTAFold can predict these interactions, advancing research into molecular pathways and cellular mechanisms.
Enzyme Engineering
For industrial applications, designing efficient enzymes is critical for processes like biofuel production or waste management. RoseTTAFold’s predictive capabilities are a game-changer for enzyme engineering.
Pandemic Preparedness
During pandemics, rapid understanding of viral proteins and their interactions with host cells is essential. RoseTTAFold can contribute to the development of vaccines and antiviral therapies by predicting viral protein structures.
Agricultural Biotechnology
RoseTTAFold is also being explored for applications in agriculture, such as designing pest-resistant proteins and improving crop yield by optimizing plant enzymes.
The Impact of RoseTTAFold on Protein Science
RoseTTAFold has sparked a paradigm shift in protein science. By significantly lowering the barriers to accurate structure prediction, it has democratized access to cutting-edge tools that were once the domain of elite institutions.
Moreover, its complementary nature to AlphaFold fosters collaboration rather than competition. Many researchers leverage both tools for their unique strengths, further advancing the field. RoseTTAFold also underscores the importance of open-source innovation in accelerating scientific progress.
The introduction of RoseTTAFold has also catalyzed global efforts to standardize protein databases, enhancing the quality of training data available for future AI-driven tools. This collective effort has strengthened the foundation of computational biology, ensuring that newer models will benefit from a more robust knowledge base.
Challenges and Future Directions
Dynamic Proteins: Predicting the conformational changes of proteins under different conditions remains difficult.
Functional Insights: Structural predictions do not always reveal the protein’s biological function, necessitating further experimental validation.
Scalability: Enhancing the model's scalability to handle larger, more complex protein systems is a critical area of ongoing development.
Data Dependency: Like all AI models, RoseTTAFold relies heavily on high-quality data for training. Addressing biases and gaps in protein databases is essential for improving their predictive capabilities.
Integration with Experimental Methods: Bridging the gap between computational predictions and experimental validations remains a priority for future research.
Real-Time Applications: Developing the capability to predict protein structures in real time, such as during disease outbreaks, is an ambitious but important goal.
Looking ahead, integrating RoseTTAFold with other AI tools, experimental data, and advanced computational platforms will likely yield even more sophisticated insights into protein science. The potential for using quantum computing to enhance predictive accuracy and processing speed also represents an exciting frontier.
RoseTTAFold is a testament to the transformative potential of AI in scientific research. By bridging computational biology with cutting-edge machine learning, the Baker Lab has provided the scientific community with a versatile and powerful tool to decode the mysteries of protein folding.
Its open accessibility and robust capabilities have not only complemented existing technologies like AlphaFold but have also opened new avenues in drug discovery, disease research, and synthetic biology. As we continue to refine and expand these tools, the future of protein science looks promising, with the potential to revolutionize how we approach some of the most pressing challenges in medicine and biotechnology.
For researchers, scientists, and enthusiasts, RoseTTAFold represents not just a technological breakthrough but a beacon of collaborative progress. Its legacy will likely inspire even more innovations in the intersection of AI and biology, pushing the boundaries of what is possible in understanding life at the molecular level.
Subscribe to our newsletter
All © Copyright reserved by Accessible-Learning
| Terms & Conditions
Knowledge is power. Learn with Us. 📚