Breaking Down Protein Structure Tools: AlphaFold, ESMFold, OpenFold, RoseTTAFold, and OmegaFold Explained?
Explore an in-depth comparison of AlphaFold, ESMFold, OpenFold, RoseTTAFold, and OmegaFold—five groundbreaking tools revolutionizing protein structure prediction. Understand their unique features, strengths, limitations, and ideal use cases in computational biology and AI-driven research.
COMPANY/INDUSTRYHEALTH/DISEASEAI/FUTUREEDUCATION/KNOWLEDGE
Sachin K Chaurasiya
12/26/20245 min read
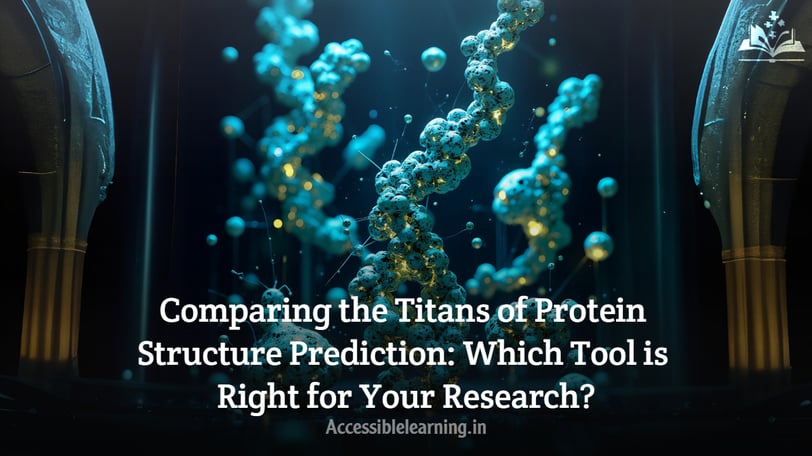
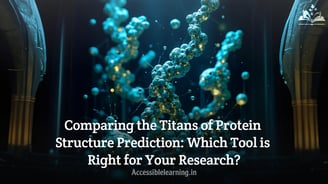
Protein structure prediction has become a revolutionary area in computational biology, driven by advancements in artificial intelligence (AI) and machine learning. Tools like AlphaFold, ESMFold, OpenFold, RoseTTAFold, and OmegaFold are leading this transformation, enabling researchers to predict protein structures accurately and accelerate breakthroughs in drug discovery, molecular biology, and genetics.
In this article, we will dive into a detailed comparison of these tools, examining their technologies, accuracy, advantages, and use cases.
AlphaFold
Developed by: DeepMind (a subsidiary of Alphabet, Google's parent company)
Release Year: 2020 (AlphaFold2, the most notable version)
AlphaFold set a new benchmark in protein structure prediction with its unprecedented accuracy. Compared to earlier tools, AlphaFold demonstrated a significant leap by achieving near-experimental precision in predicting 3D protein structures, as showcased during the CASP14 competition. Traditional methods like homology modeling and ab initio predictions often fell short for proteins with no known templates or complex folds, whereas AlphaFold's deep learning-based approach overcame these limitations by leveraging massive datasets and advanced attention mechanisms. It uses deep learning to predict 3D structures of proteins based on amino acid sequences. AlphaFold2's success in the Critical Assessment of Protein Structure Prediction (CASP14) demonstrated its ability to solve structures with near-experimental accuracy.
Key Features
Utilizes deep neural networks and attention mechanisms to analyze protein sequences.
Integrates evolutionary information from multiple sequence alignments (MSA).
Generates highly accurate 3D models of protein structures.
Includes confidence scores to measure prediction reliability.
Strengths
Accuracy close to experimental methods like X-ray crystallography.
Free access to the AlphaFold Protein Structure Database, which contains over 200 million protein structures.
Accelerates drug discovery and protein engineering.
Limitations
Requires high computational power for training.
May struggle with disordered proteins or highly dynamic protein structures.
Use Cases
Structural biology research
Drug design and molecular docking
Understanding protein-protein interactions
ESMFold
Developed by: Meta AI (formerly Facebook AI)
Release Year: 2022
ESMFold leverages language models trained on protein sequences to predict protein structures efficiently. Unlike AlphaFold's deep learning approach, which incorporates multiple sequence alignments (MSAs) and evolutionary data to enhance accuracy, ESMFold uses transformer-based language models that analyze patterns within protein sequences themselves. This innovation allows ESMFold to bypass the computationally intensive step of generating MSAs, making it faster and more accessible while still achieving competitive accuracy. Unlike AlphaFold, it does not rely heavily on evolutionary information or multiple sequence alignments, making it faster while maintaining competitive accuracy.
Key Features
Powered by Evolutionary Scale Modeling (ESM), a large-scale protein language model.
Does not require MSAs, reducing computational requirements.
Capable of ultra-fast protein structure prediction.
Strengths
Faster prediction times compared to AlphaFold.
Lightweight and accessible for researchers with limited resources.
Suitable for predicting protein structures in novel, low-knowledge proteins.
Limitations
Slightly less accurate than AlphaFold for complex proteins.
May lack precision in predicting protein structures that benefit from evolutionary information.
Use Cases
High-throughput structure prediction for large protein datasets.
Genomic and proteomic studies.
Rapid exploration of unknown protein sequences.
OpenFold
Developed by: Open-source community, led by Columbia University and others
Release Year: 2022
OpenFold is an open-source implementation of AlphaFold, designed to increase accessibility and transparency. Its open-source nature has been particularly impactful in academic and research environments, where institutions have leveraged OpenFold to customize workflows, integrate it into bioinformatics pipelines, and train new models on specialized datasets. For example, labs with limited access to proprietary software have used OpenFold to explore protein structures in novel organisms or synthetic proteins, fostering collaboration and innovation within the scientific community. It replicates AlphaFold's architecture and performance while providing researchers with a customizable framework.
Key Features
Open-source codebase for full flexibility.
Matches AlphaFold2's accuracy and methodology.
Reduces reliance on proprietary models.
Allows integration with other tools for customized workflows.
Strengths
Transparent and fully accessible for research and academic purposes.
Replicates AlphaFold's performance while offering greater adaptability.
Community-driven improvements enable rapid innovation.
Limitations
Requires significant computational resources to run effectively.
Training from scratch may be challenging for resource-constrained labs.
Use Cases
Research requiring transparency and code flexibility.
Custom integration into bioinformatics pipelines.
Open collaboration projects in computational biology.
RoseTTAFold
Developed by: University of Washington's Institute for Protein Design (IPD)
Release Year: 2021
RoseTTAFold is a deep learning-based tool that integrates protein structure prediction and protein-protein interaction modeling. Unlike other tools, it employs a three-track neural network architecture that uniquely processes and integrates sequence information, pairwise distances, and spatial coordinate data simultaneously. This approach allows RoseTTAFold to build a more comprehensive understanding of the interplay between sequence and structural constraints. By leveraging this architecture, it excels at multitask learning, enabling accurate predictions for both single-protein structures and protein-protein interaction complexes. Moreover, its ability to infer multi-chain protein dynamics has made it particularly useful in studying large complexes such as ribosomes or virus capsids.
Key Features
Three-track architecture for efficient multitask learning.
Predicts both single-protein and protein-protein interaction structures.
Faster than AlphaFold, though slightly less accurate.
Strengths
Excellent for multi-chain protein complexes and interactions.
Lightweight compared to AlphaFold.
Open-source availability.
Limitations
Slightly lower accuracy than AlphaFold for single protein structures.
Limited adoption compared to AlphaFold.
Use Cases
Modeling protein complexes and interactions.
Structural prediction in molecular biology research.
Understanding protein folding dynamics.
OmegaFold
Developed by: Helixon (AI-based biotech company)
Release Year: 2022
OmegaFold stands out by using single protein sequences (without MSAs or evolutionary data) to predict structures. This approach is particularly advantageous in scenarios where evolutionary data is unavailable or insufficient, such as for orphan proteins, synthetic sequences, or proteins from organisms with limited genomic data. For instance, researchers exploring newly discovered proteins in extremophiles or designing novel proteins in synthetic biology can benefit greatly from OmegaFold's MSA-free methodology. This makes it ideal for de novo protein prediction, especially for proteins lacking homologous sequences in databases.
Key Features
MSA-free structure prediction, reducing computational complexity.
Focuses on sequence-only inputs to predict structures.
Optimized for orphan proteins and novel sequences.
Strengths
Excellent for predicting proteins with no evolutionary context.
Fast and efficient compared to AlphaFold.
Enables de novo protein structure prediction.
Limitations
Less accurate than AlphaFold when MSAs are available.
Still developing its adoption in research communities.
Use Cases
Orphan protein studies.
Predicting structures in genomic and synthetic biology.
Computational protein design.
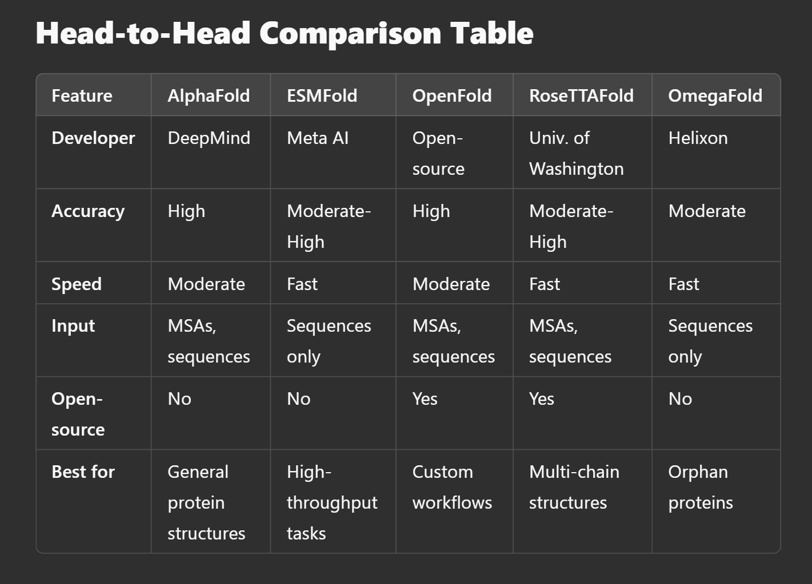
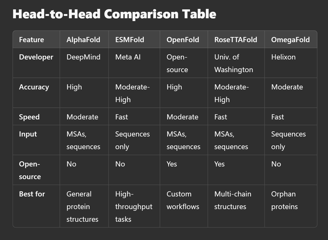
The choice of protein structure prediction tool depends on your specific needs:
AlphaFold: Best for high-accuracy predictions, particularly when evolutionary data is available.
ESMFold: Ideal for speed and efficiency without relying on MSAs.
OpenFold: A perfect open-source alternative for customizable workflows.
RoseTTAFold: Great for protein-protein interactions and complex modeling.
OmegaFold: Best for de novo structure predictions with no evolutionary data.
Each tool has its strengths and limitations, and often a combination of these approaches can yield the most comprehensive results. Researchers, bioinformaticians, and biotechnologists now have a diverse toolkit to decode the complexities of proteins and accelerate discoveries in medicine and biology.
Subscribe to our newsletter
All © Copyright reserved by Accessible-Learning
| Terms & Conditions
Knowledge is power. Learn with Us. 📚